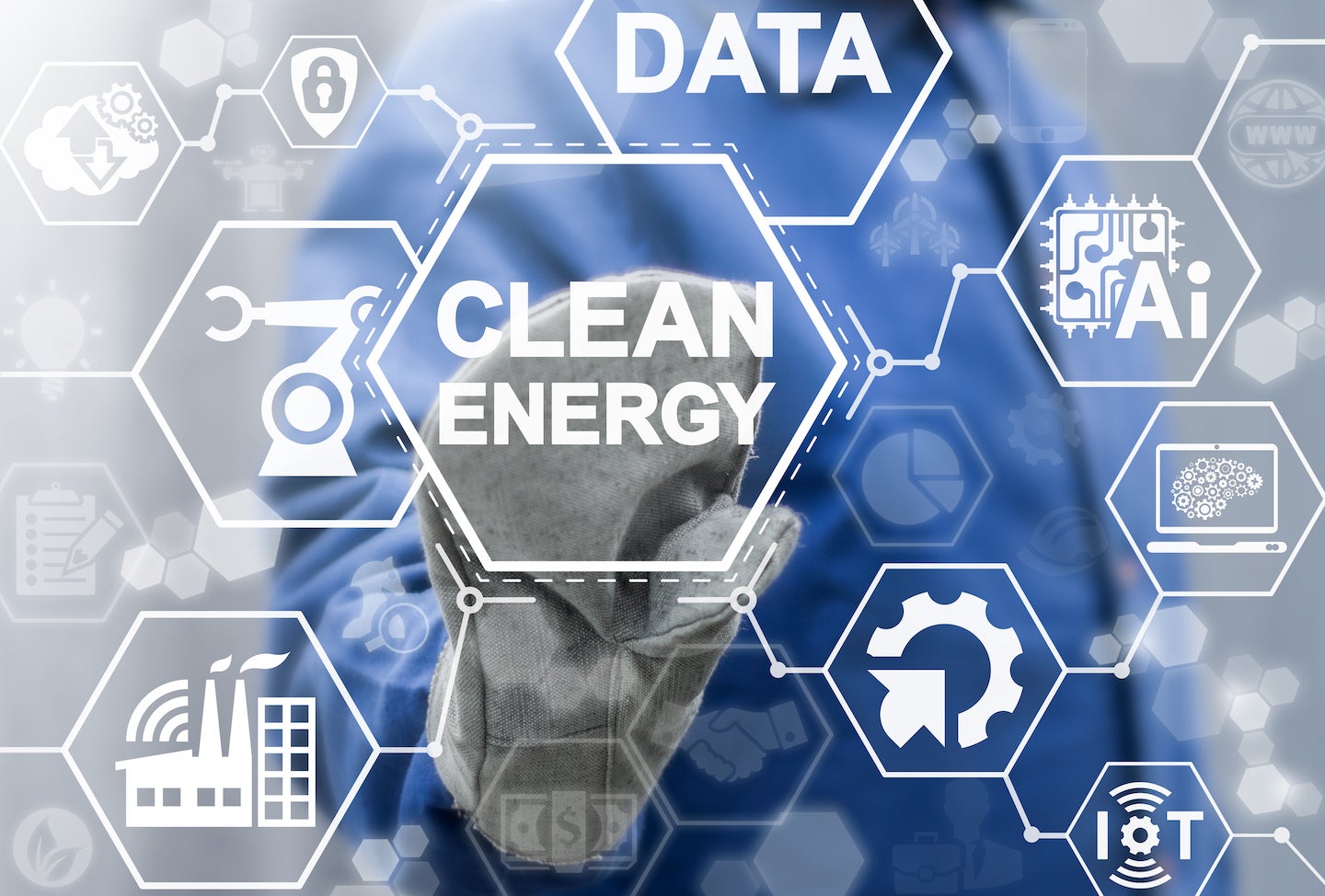
Nuclear science, towering 200m power plants, and millions of miles of electrical cable are some of the things making the power industry one of the most process driven on Earth. Power companies at every stage of the value chain must make decisions regarding how to best use and maintain their assets to balance supply with demand. They are therefore natural candidates for the integration of AI, which has the potential to enhance generation, transmission, distribution, and the experience for end users.
The power industry is often considered as being stuck in its ways. Not out of stubbornness, but due to the difficulty in digitally transforming age-old infrastructure and training an ageing workforce. The question is therefore how can power companies integrate AI into their business models?
Equipment and engineering
AI implementation in the power industry starts with equipment. Most power plants and electricity grids typically have lifespans extending decades. Therefore, while new AI-powered equipment can act as a key enabler, most power companies simply use add-ons such as sensors, probes, meters, and thermal imaging equipment to collect real-time data, and third-party AI software to process this data. This practice is commonly known in the industry as predictive maintenance.
AI-based predictive maintenance algorithms and software can detect faults and repair them before an asset breaks down. Industrial automation companies such as Emerson, ABB, and GE are leaders in providing predictive maintenance solutions such as vibration monitoring, infrared thermography, and lubricant oil analysis.
Harnessing the lifeforce of stars
Some of the most exciting AI developments in power come in the field of nuclear energy, which attracts attention due to its high energy density and low emissions profile. Applications of AI in nuclear fission revolve around predictive maintenance of equipment and the safer disposal of radioactive waste. However, AI has the most potential to enact change in nuclear fusion. The emerging energy source is largely still in the research & development phase, with few industry experts expecting the availability of commercial fusion reactors before 2030. Research groups often share fusion reactors, limiting the time available for experiments. AI can speed up the R&D timeline through modelling and simulations. In February 2022, DeepMind collaborated with the Swiss Plasma Center (SPC) to develop a deep reinforcement learning system for tokamak nuclear fusion simulations.
See Also:
How does generative AI fit into the picture?
The potential for generative AI to disrupt the power industry is large, despite there currently being no prominent examples of its use.
How well do you really know your competitors?
Access the most comprehensive Company Profiles on the market, powered by GlobalData. Save hours of research. Gain competitive edge.
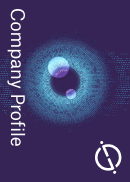
Thank you!
Your download email will arrive shortly
Not ready to buy yet? Download a free sample
We are confident about the unique quality of our Company Profiles. However, we want you to make the most beneficial decision for your business, so we offer a free sample that you can download by submitting the below form
By GlobalDataThe most natural application of generative AI in the power industry is facilitating the interpretation of the massive amounts of data coming from predictive maintenance equipment. AI algorithms are already being used to analyse this data, but generative AI capabilities like large language models can help reduce the barriers to understanding the technology for staff that lack the required skills to use existing AI systems. In July 2023, ABB and Microsoft announced a partnership to integrate Copilot, Microsoft’s large language model, into Ability Genix—ABB’s IoT, analytics, and AI platform.
Unsurprisingly, generative AI has the most value at the edge of the power value chain, using natural language processing to help users make more optimized decisions about their energy consumption. Smart home energy systems currently use AI and machine learning algorithms to analyse energy usage patterns. Although no prominent generative AI models exist specifically for use in managing energy usage, the technology can reduce the barriers to educating end users on energy logistics as well as creating effective strategies for energy consumption. In this way, homeowners can reduce consumption and save money on their energy bills.
Related Company Profiles
ABB Ltd
SPC GmbH
CoPilot LLC
Emerson LLC
GE Co Ltd