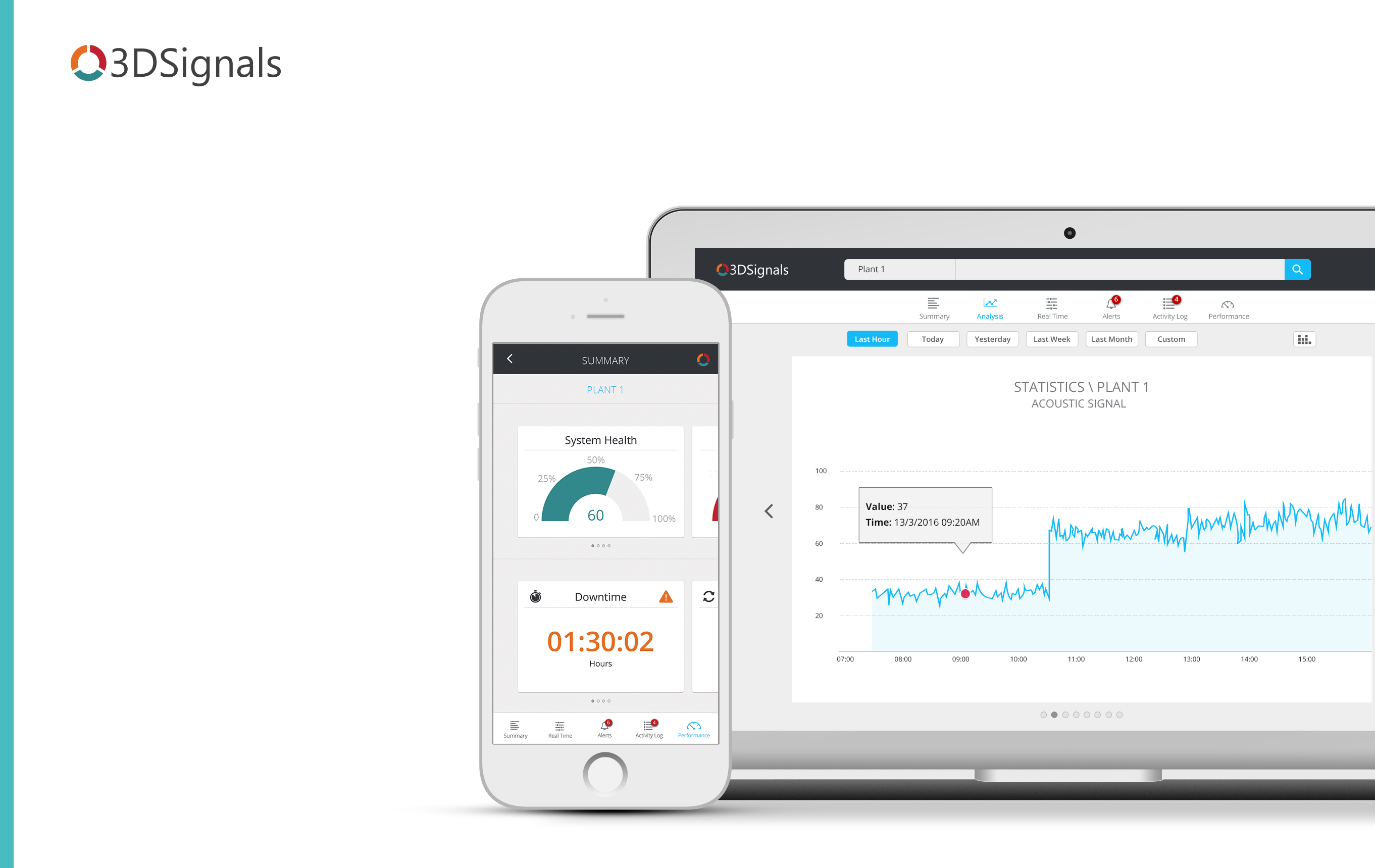
A new technology that uses airborne ultrasonic sensors to detect anomalies in the sounds machines make while running is already saving power companies hundreds of thousands of dollars by preventing unpredicted downtime.
Unlike other acoustics-based maintenance solutions, which either call for technicians to go from machine to machine, sampling and diagnosing problems, or require complex integrations, training and retrofitting of old industrial assets, Israeli start-up 3D Signals’ system allows for accurate and reliable coverage of a wide variety of equipment with a small number of cheap sensors.
As the company’s co-founder and CEO, engineer Amnon Shenfield, summarises: “Energy production must operate reliably in dynamic demand conditions, and we help guarantee that equipment runs smoothly while maintenance technicians and engineers can focus solely on the machinery that needs it most and not waste valuable time and resources conducting unnecessary inspection and maintenance on healthy equipment.”
Looking ahead, with the predictive maintenance market set to reach $11 billion by 2022, driven largely by IoT technology, the potential of the system – both to be integrated directly into new industrial machines and retrofitted to older ones – is huge.
Elly Earls meets Shenfield to find out how the technology works and why he believes he can democratise sound as a meaningful tool for predictive maintenance, operational efficiency and safety applications.
Elly Earls: Tell me a bit about how the company started.
Amnon Shenfield: The idea for 3DSignals was born on a train ride. I was riding a train in the UK when suddenly there was a loud alarming noise – one that made everyone stop what they were doing and wonder if they should hold onto something.
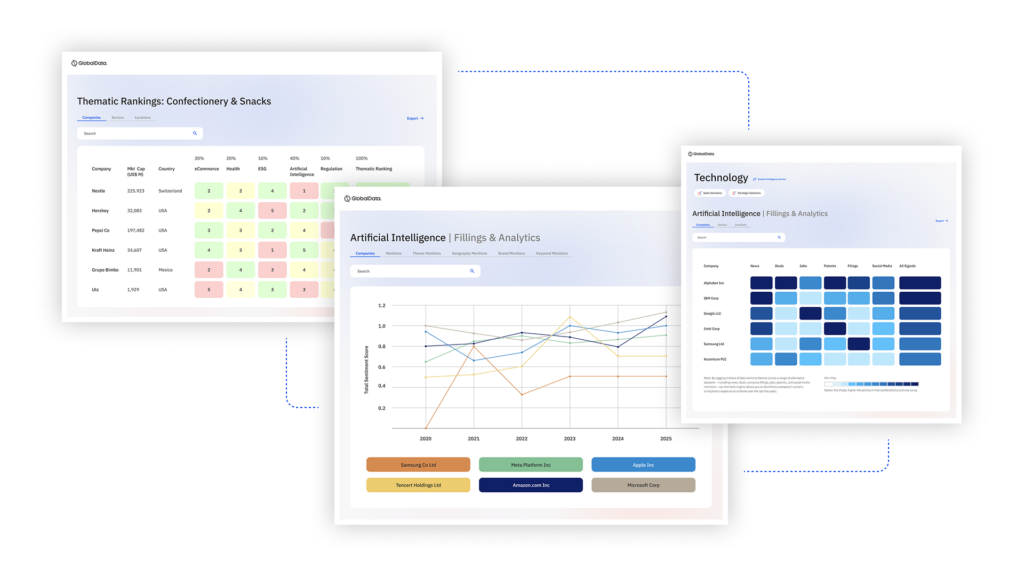
US Tariffs are shifting - will you react or anticipate?
Don’t let policy changes catch you off guard. Stay proactive with real-time data and expert analysis.
By GlobalDataThe noise passed and everything was okay, but it made me think that if an experienced train engineer had been there, he or she would probably be able to tell almost instantly from the noise what the problem was, and most importantly whether it was a sign of danger, and how to fix it after we had reached our destination.
So together with three friends (all experienced engineers), we started to work on this idea around airborne acoustics for predictive maintenance.
EE: How long did it take to get the company off the ground and what were some of your early big wins?
AS: We were fortunate to get some good momentum early on. After developing the technology, we began to test it in a steel production plant that was innovative enough to allow us to experiment around their heavy machinery.
It didn’t take very long for the system to detect meaningful anomalies and save the factory hundreds of thousands of dollars by preventing unpredicted downtime. Six months after beginning the experiment, we quit our jobs and formed the company 3DSignals with our first design partner.
EE: How does your technology work to detect anomalies and predict when equipment will fail?
AS: Predisound™ is our proprietary hardware and software system that includes airborne ultrasonic sensors that detect anomalies in the sound that machines make while running. The platform’s deep learning and predictive analytics software processes these sound signals and aggregates the data, allowing authorised users to remotely monitor their machines from any location and receive alerts on changes in sound patterns.
The modern technologies we’re bringing to the table include ultrasonic sensors developed specifically for harsh industrial environments, as well as innovative deep learning predictive analytics software that learn to recognize and alert on specific acoustic signatures which identify various failure states such as bearing friction, shaft misalignment, blocked filters, lack of lubrication, and so on.
EE: Can you give me an example of the technology in action within the power industry?
AS: In a traditionally very conservative sector, which is famously difficult to penetrate due to its highly standardised and regulated work procedures, Enel Green Power’s innovation department has “opened the door” and given us access to begin piloting our acoustic deep learning technology in hydroelectric power plants to monitor turbines, generators and peripheral support equipment such as valves and pumps.
Within three weeks from the installation of our sensors in the first pilot plant, 3DSignals identified a significant operational anomaly that no other monitoring system, out of several that had previously been installed at the plant, identified.
Furthermore, due to the nature of airborne acoustic signal propagation and intuitive understanding of such signals by experienced engineers, the dispatched maintenance crew identified the failing component causing the operational anomaly within 10 minutes from arriving to the plant to investigate the incident. These results have been unprecedented.
Fuelled by this early success, we have since continued to deploy our acoustic monitoring deep learning systems in additional plants with Enel and are jointly looking to extend the relationship further to additional plants and equipment types. Our goal is to assist Enel’s Operational and Maintenance team prioritise work and understand hidden ties between operational procedures and equipment deterioration rates.
EE: Why would you advise other power companies to invest in your technology?
AS: Condition monitoring via acoustics has four major benefits. The ability to monitor remotely – anywhere, anytime. Secondly, early detection: As changes begin to occur in rotating, mechanical equipment, the subtle nature of ultrasound allows these potential warning signals to be detected early, before actual failure, often before they are detected by vibration, temperature and audible means.
Third is greater coverage with fewer sensors: Acoustic sensors are more economical as a single sensor can cover anywhere from a single component to an “orchestra” of machines, compared with multiple, sometimes tens of, sensors per each piece of equipment. And fourth, we believe that by using our technology, companies in the industrial and commercial sectors are actually investing in themselves. Operational downtime is a huge drain on human and financial resources. This is why Enel, which is in the business of energy production and distribution, finds such value in our technology. There is of course an investment with technology like ours, but companies should also consider the long-term return on that investment
EE: How fast do you anticipate the power sector will catch on to the potential of your technology?
AS: We actually think that the IoT hype cycle is still in its early stages in many ways. While there’s a lot of excitement about the potential to apply data-driven algorithms to large data streams from industrial assets, IoT still has a while to go before hitting a mainstream productivity plateau.
One of the main insights we’ve gleaned from the space so far is that there are still data challenges that need to be overcome to push IoT predictive maintenance technologies into mainstream adoption and success. The first issue is around data quality [and] the difficulty [in] obtaining high-quality labelled data from industrial machines to begin with. Secondly, it’s then even more challenging to then apply that data to provide human engineers and technicians with relevant and actionable condition-based maintenance insights.
Much of the data quality challenge will be addressed by new deep learning algorithms that mimic the human brain and can be used to build more accurate predictive models. These models will be able to apply insights from previously labelled data to new unlabelled data so that both predictive and prescriptive analysis will become more accurate over time.
That said, advancements in Industry 4.0 technologies such as the deep learning powered airborne acoustic system we developed will increasingly play a larger role in the power industry and adoption will only continue to accelerate as the tangible benefits are realised more and more.